点击次数:
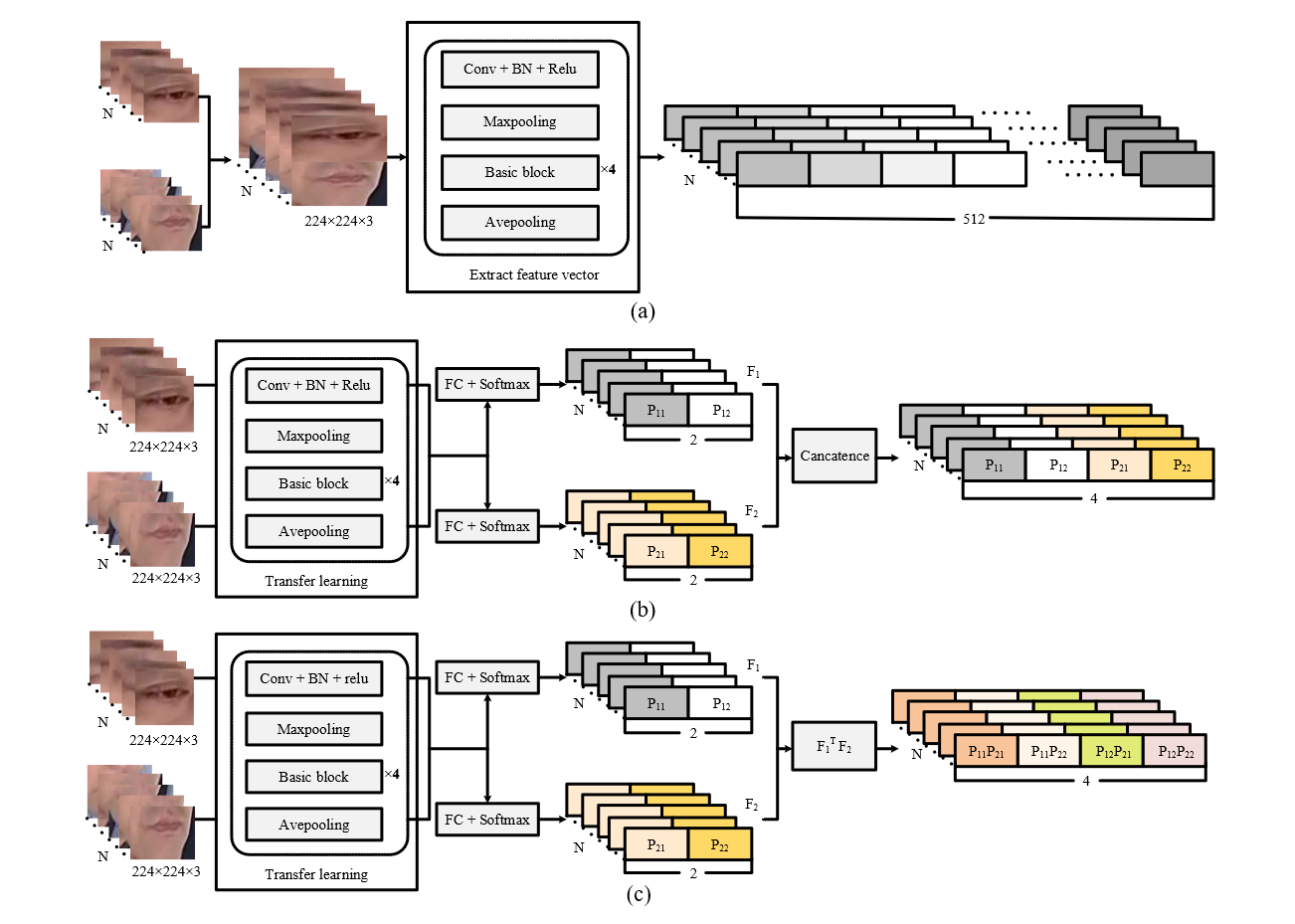
影响因子:2.4
DOI码:10.3390/math9222908
发表刊物:Mathematics
关键字:open-pit truck; driver fatigue; feature coding; LRCN
摘要:Due to complex background interference and weak space–time connection, traditional driver fatigue detection methods perform poorly for open-pit truck drivers. For these issues, this paper presents a driver fatigue detection method based on Libfacedetection and an LRCN. The method consists of three stages: (1) using a face detection module with a tracking method to quickly extract the ROI of the face; (2) extracting and coding the features; (3) combining the coding model to build a spatiotemporal classification network. The innovation of the method is to utilize the spatiotemporal features of the image sequence to build a spatiotemporal classification model suitable for this task. Meanwhile, a tracking method is added to the face detection stage to reduce time expenditure. As a result, the average speed with the tracking method for face detection on video is increased by 74% in comparison with the one without the tracking method. Our best model adopts a DHLSTM and feature-level frame aggregation, which achieves high accuracy of 99.30% on the self-built dataset.
论文类型:期刊论文
学科门类:工学
一级学科:矿业工程
文献类型:J
卷号:9
期号:22
页面范围:2908
字数:6000
是否译文:否
发表时间:2021-11-15
收录刊物:SCI